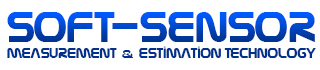
Soft-Sensor (Virtual-Sensor) technology
1. Introduction 2. Solving industry problems 3. Technology 4. References1. Introduction
Instrumentation providing accurate measurements of system variables is at heart of each process or machine operation. Often affordability and quality of those measurements limit system's performance or product quality, as the available instrumentation is frequently too expensive or difficult to install or simply not realiable or accurate enough.
Soft-Sensors, also known as Virtual-Sensors, use system knowledge and avaliable measurements in order to improve availability, realibility, accuracy and cost of measurements of the variables of interest.
This is possible due to application of numerical models and state of the art digital filtering techniques. The software acceses and analyzes available measurements of variables correlated with the variables of interest. Soft-Sensors are implemented: 1) as software integrated into the current data aquisition and control system, using installed instrumentation and computing power or 2) as independent physical device.
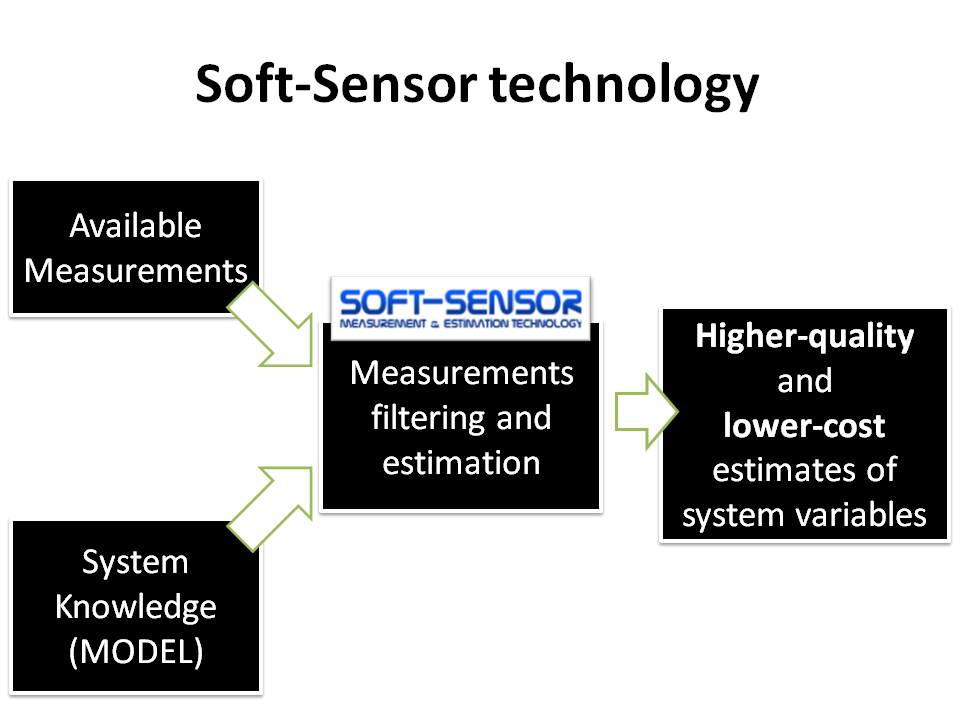
2. Solving industry problems
Soft-Sensors provide solutions for a number of problems encountered in the industry, related to availability, cost, quality and realibility of measurements. Improved measuresements allow for better quality of product or system performance through improved process control, operation and fault detection.Measurements affordability and availability
Soft-Sensor provides estimates of process variables of interest in situation where those variables cannot be measured at all or frequently enough using classical instrumentation due to economical or technological limitations, like lack of space, harsh environmental conditions, extreme operational conditions. Those estimates allow to solve problems like the following.
Problem 1: measurement is very expensive and thus it is performed only periodically.
Solution: Soft-Sensor provides continious estimates of the variable.
Problem 2: instrumentation is too expensive and has not been installed, making the process more difficult to control.
Solution: Soft-Sensor provides cheap estimates of the approximate values of variables improving the operation.
Problem 3: no instrumentation exists that can directly measure the process variables of interest, e.g. heat load into a system.
Solution: Soft-Sensor provides estimates of the variables, using available measurements, e.g. combining the knowledge of system dynamics and measurements of coolant mass flow rates and system temperature to calculate the heat load.
Problem 4: no instrumentation can be installed at the location of interest due to harsh conditions or simply lack of space, e.g. no place for thermomether at the location of interest.
Solution: Soft-Sensor provides estimates of the variables, using available measurements taken at different locations, that are correlated to the one of interest, e.g. using the knowledge of the temperature around the point of interest and the system model allows to estimate that at the location of interest.
Measurements accuarcy and reliability
Adding the information about the system to the available measurements is a simple method to improve the accuracy, realiablity and fault detection of the data aquisition. Combining information from multiple types of sensors allows to combine strenghts of different technologies and reduce their weaknesses. Redundancy is introduced into the measurment system using multiple instruments measuring correlated values, so that the failure of one or multiple instuments wont stop a Soft-Sensor from delivering a meaningful data. Problem 1: two instrument exist to measure the variable of interest, one delivering precise but biased measurements and other characterized by much less precision but having no bias - none provides the desired accuracy.
Solution: Soft-Sensor merges the measurements coming form both instruments to provide precise and unbiased estimate of the measured variable.
Problem 2: the measurement is critical for system operation and the instrumentation is known to fail or is affected by large errors during some phases of operation (e.g. start up), the availablility of measurement must be improved
Solution: Soft-Sensor merges the measurements coming form multiple instruments measung correlated data introducing the redundancy and thus increasing the measuremnt relibility and easing the fault detection.
3. Technology
Soft-Sensors need measurements, models and estimation algorithms to work.
Measurements
Due to the limitless possible realizations of the Soft-Sensor technology, the exact demand on the hardware needed is studied case by case. Taking advantage of the system knowledge may allow to use more affordable instrumantation to obtain the measurements of desired accuracy. Soft-Sensor may share some of the existing hardware capacity.
Models
Models used in Soft-Sensors represent the knowledge of the system that is exploited to improve the quality of measurements. The models complexity needed varies depending on the goals of the Soft-Sensor development and the complexity of the system, ranging from none to thousands of dynamic variables.
The simpler models need very little computing power to execute the estimation algorithms. In case of more complex models, installing additional computing power may be necessary. Many models allow for implementations as embeeded systems.
The models may be based on: 1) the knowledge of the physical processes governing the beahviour of the process variables of interest - so called first principles models or white-box models, or 2) identified from the sytsem's operational data with little insight into the system structure - so called black-box models, or 3) mixed grey-box models merging the two previous approaches. At the Soft-Sensor, we are specialized in deveolping of first principles thermo-hydraulic and kinetic models for process and aeronautical industries.
Estimation algorithms
Similarily as in case of the models, wide range of possible estimation algorithms may be applied to calculate the variables of interest. Starting with simple linear filering techniques like Lueneberger Observer or Kalman Filter and ending with state of the art non-linear estimation algorithms like Extended Kalman Filter or Moving Horizon State Estimation, extending applicability of Soft-Sensors to challanging, highly non-linear systems operated at frequently changing operating points.
4. References
Non-linear Moving Horizon State Estimation and Control for the Superfluid Helium Cryogenic Circuit at the Large Hadron ColliderSoft-Sensor is a start-up project focused on providing reliable and affordable advanced controls functonality. We are actively looking for potential clients and are open for a wide range of projects, ranging from estimation, through fault detection to control. The work is led by Rafal Noga who has been working on advanced non-linear control solutions for process industry since 2007. In his realisations of Model Predictive Control and Moving Horizon Estimation, Rafal has been applying non-linear first principles models and tailored optimization algorithms.
We are located in Grenoble, France. For more information in English, German, French, Spanish and Polish, call us at +33 604 413 827 or send us an email at .